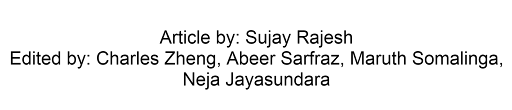
Introduction
Humans have identified, synthesized, and even tested millions of molecules thus far. However, out of the near-infinite possibilities, only a small fraction of molecules have been discovered thus far. But what if it was possible to exponentially accelerate this process?
Current System
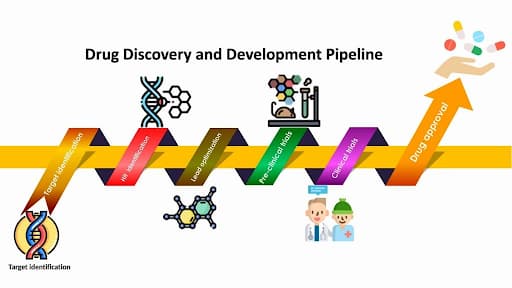
Generally, drug discovery is a long, arduous process; while thousands of molecular compounds and existing treatments are tested on a disease based on new insights, barely any are chosen for additional analysis. More specifically, approximately 10,000 drugs are tested, on average, in the discovery stage. Of this, around 250 continue to the preclinical stage, and only about 5 are deemed ready for further analysis in the clinical trials.
Therefore, with this current process, there is an approximate 0.05% chance of discovering a possibly effective drug. Even so, of the scarce amount that pass to the clinical trials, even fewer advance beyond that stage to reach commercial availability. With an average success rate of drugs in clinical trials at approximately 11.1%, it can be reasonably inferred that a staggeringly low 0.005%, or 1 in 20,000 molecules tested become an actual product.
Despite the current model’s inefficiency, until very recently there were no better alternatives. To put it into perspective, the US Food and Drug Administration (FDA) currently uses the previously described selective method. In the past few years, a more productive option has begun to emerge – artificial intelligence.
Digital Chemistry
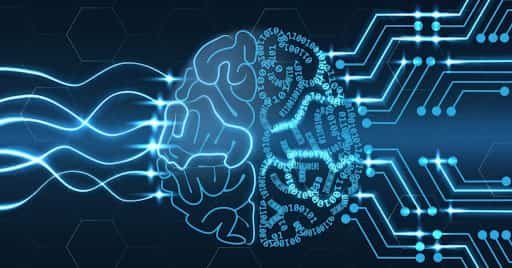
In a 2022 MIT study, a machine learning model was trained to suggest molecular structures that have certain properties and can be synthesized. By feeding the model with a multitude of molecules, elements, and reactions that take place to form its structure, the model learns to synthesize desired molecules independently. The trained model is then inputted with a set of “building blocks” to create a molecule (list of chemicals and their possible reactions) to restrain the search to what is possible; then, a decision tree is employed to find the final molecule. Although the model is still in development, this methodology could revolutionize the drug discovery process, and open up doors for limitless innovation in the future.
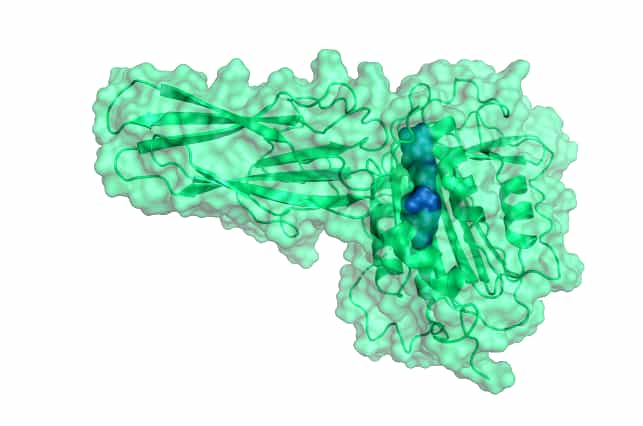
One company that has also worked on utilizing artificial intelligence in drug development considerably, and currently holds the title for the “leading computational platform for molecular discovery and design” is Schrödinger. With a working procedure for molecular discovery, and several patents, this company has developed molecules that have passed the preclinical stage, and could likely be effective in treating diseases. For example, the drug SGR-1505 blocks a protein called MALT1, which is involved in growth of B-cell lymphomas (cancers of the immune system) and therefore, could be a promising way to treat those cancers. This drug was discovered through machine learning methods, and serves as a testament to the extent of the possibility in this field.
Conclusion
Traditional drug discovery, although foundational, is an extremely tedious and inefficient process that has considerable potential for improvement. To reduce the time and resources involved with this system, using machine learning procedures can significantly reduce the effort necessary to successfully achieve molecular discovery. Despite the old system still being in use, digital chemistry’s integration of machine learning and AI can potentially redefine the field entirely – a new era.
Sources:
- https://news.mit.edu/2022/ai-molecules-new-drugs-0426
- https://www.schrodinger.com/platform/
- https://www.schrodinger.com/pipeline/malt1/
- https://www.schrodinger.com/pipeline/
- https://ftloscience.com/ai-in-drug-discovery-chemical-synthesis/
- https://www.fda.gov/patients/drug-development-process/step-1-discovery-and-development
- https://www.cardinalpeak.com/blog/best-practices-when-training-machine-learning-models
- https://meilerlab.org/drug-discovery-design/
Add a Comment